Post-event flood mapping for road networks using taxi GPS data
In this study, the researchers propose a methodology that leverages taxi GPS data to support post-event flood mapping for the road network. Dynamically updated and fine-grained flooding maps are critical for situational awareness and decision support. However, traditional methods, such as eyewitness reports, remote sensing, and hydrology models, may fail to correspond with the rapidly changing urban hydrological environment. The presence of crowdsourced data (such as social media data) allows for timely and cost-effective monitoring of flood hazards through collective observations; however, such data can be unreliable due to sample bias and low spatiotemporal resolution. Therefore, new measures to identify flood-affected roads are desirable.
This method can identify whether a significant reduction in the taxi passing rate for a road segment was related to precipitation, and automatically recognize the flood-affected roads based on a logistic regression model. Using taxi GPS data in Shenzhen as an example, the researchers derived the flood map of the road networks, and compared and validated the results. This study demonstrated the usefulness of taxi GPS data in generating high-quality flood maps and the value of incorporating multiple data sources for sensing near real-time flood in the city.
Explore further
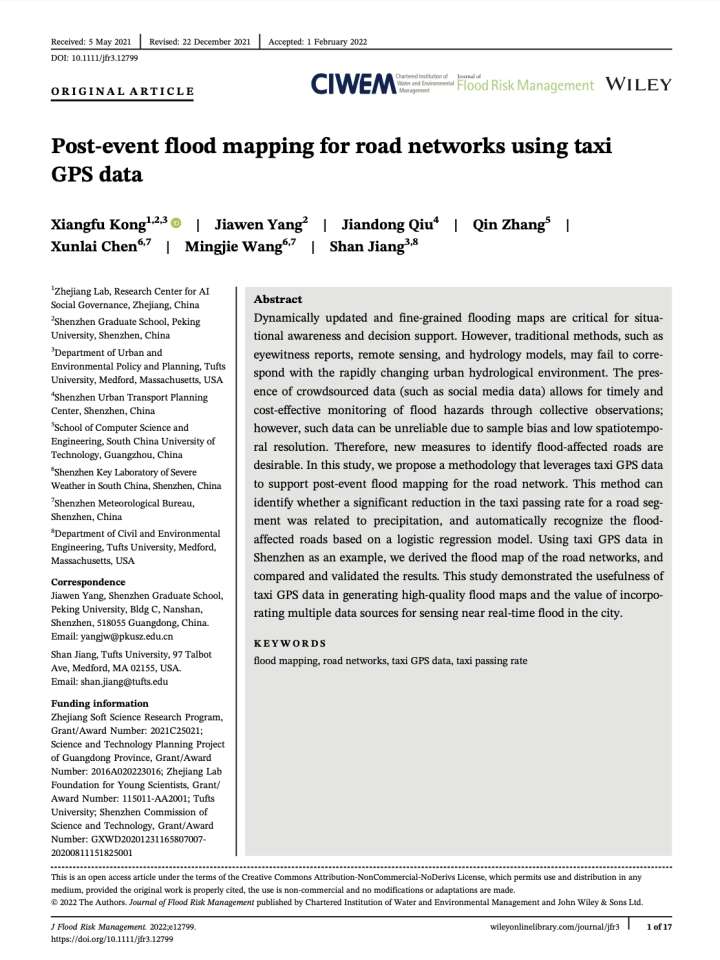