A versatile suite of computational models, already used to forecast magnetic storms and potential power grid and telecommunications disruptions, is preparing to welcome a larger group of users.
By Tuija Pulkkinen, Tamas I. Gombosi, Aaron J. Ridley, Gabor Toth, and Shasha Zou
Just as light from the Sun drives Earth’s seasons and day-to-day weather, streams of plasma and other particles from the Sun interact with Earth’s magnetic field and upper atmosphere, creating aurorae and other phenomena referred to as space weather. Solar wind–induced magnetic storms also cause current surges in electrical power lines, disruptions in telecommunications, and radiation damage to satellites.
Severe space weather could disrupt critical elements of the nation’s infrastructure, with highly damaging effects to commerce and national security. This hazard was recognized in the recent Promoting Research and Observations of Space Weather to Improve the Forecasting of Tomorrow (PROSWIFT) Act, signed into law on 21 October 2020. Because space weather affects systems in space and on the ground—and communication between the two—developing protective measures requires understanding relevant physical processes in the space environment.
Here we describe our efforts to develop the University of Michigan’s Space Weather Modeling Framework (SWMF), which not only contributes to scientific understanding of space weather processes but is also used for space weather forecasting and issuing hazard alerts. The SWMF is the product of a large multidisciplinary collaboration among model developers and users, and this community is now preparing to become even more inclusive by releasing a major part of the SWMF as a full-fledged open-source distribution.
A Model Suite That Integrates Global and Local Scales
The space weather environment encompasses the Sun, the solar atmosphere, and the solar wind, which interacts with Earth’s magnetosphere, ionosphere, and upper atmosphere. Currently, we cannot satisfactorily predict near-Earth solar wind conditions starting from observations of the Sun. Therefore, current space weather forecasting relies on measuring the incoming solar wind upstream of Earth (typically about 1 hour in advance) and modeling potential responses in geospace (comprising the upper atmosphere, ionosphere, and magnetosphere).
The SWMF describes each part of geospace using different types of physical models (Figure 1) and then couples the models together to form a self-consistent description of the Sun-Earth system. In a standard configuration, three models are used to represent conditions in geospace. A computationally efficient fluid (magnetohydrodynamic (MHD)) model describes the near-Earth solar wind and global magnetosphere. A more detailed kinetic model accounts for the large range of energies and complex particle distributions present in the inner magnetosphere, which is threaded by closed magnetic field lines tied to Earth at both ends. And an electrostatic model covers the ionized upper atmosphere. This suite of models is accurate and computationally efficient enough that it is used operationally by NOAA’s Space Weather Prediction Center (SWPC) to inform forecasters who issue warnings and short-term predictions of impending space weather conditions.
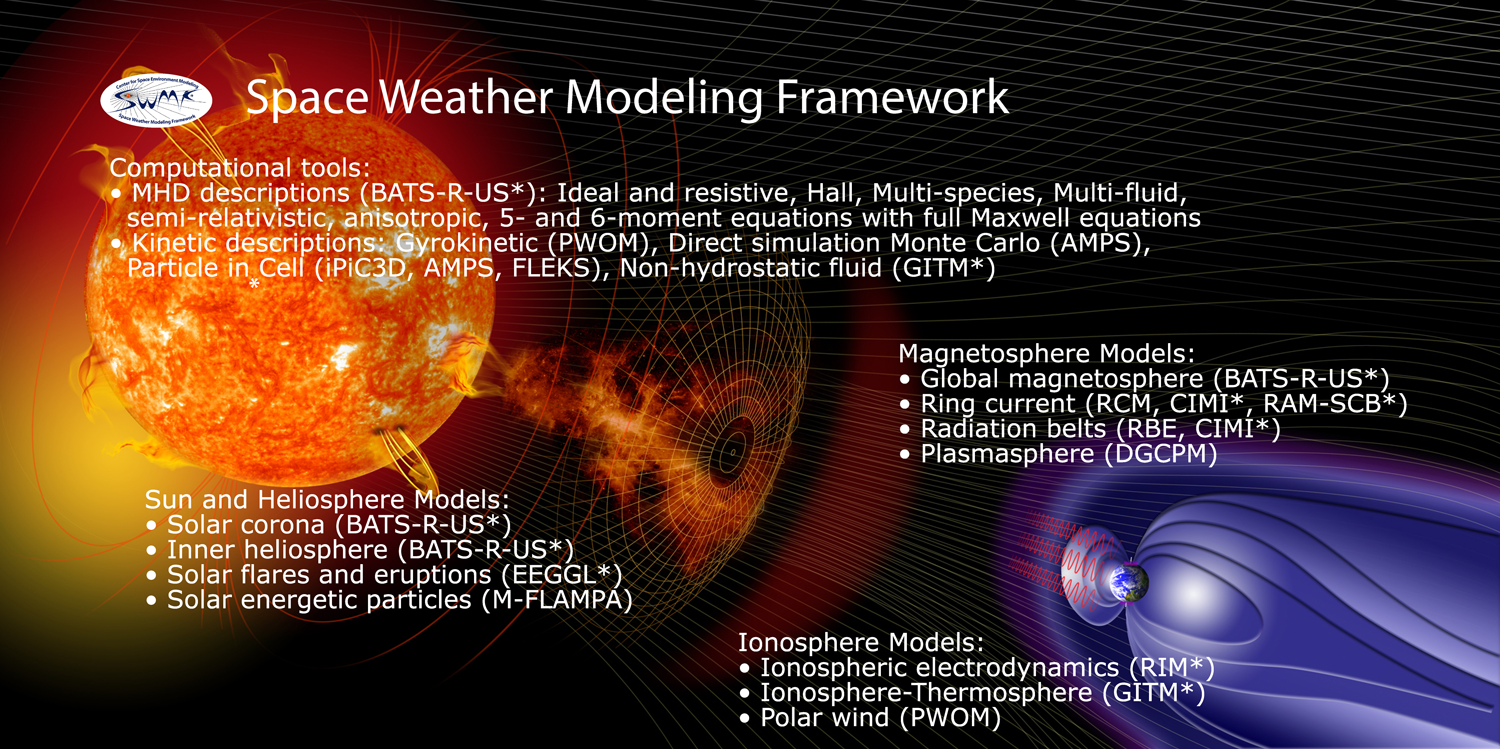
Optimizing Competing Priorities
As with all models of complex systems, space weather models are computationally intensive, and various assumptions and simplifications may be introduced to accommodate limitations in computing resources. However, oversimplification can produce inaccurate results. It is for that reason that over the past 3 decades, the research community has challenged the use of the relatively simple MHD models to describe complex processes that undoubtedly include nonlinear and small-scale physics.
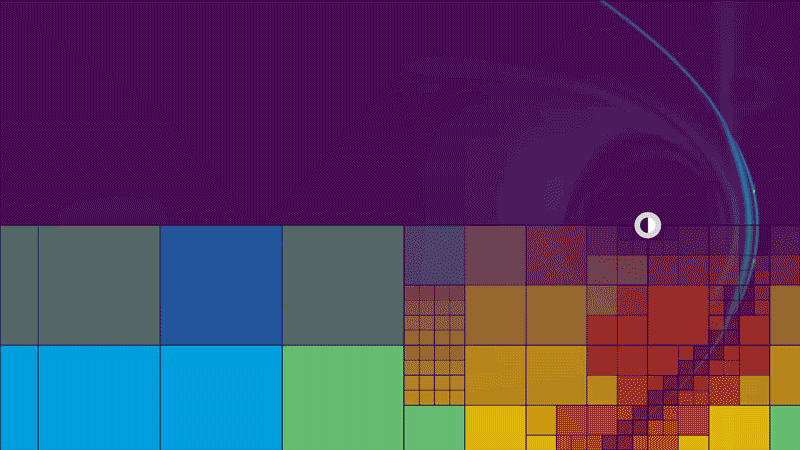
The SWMF tackles the contrasting needs for accuracy and computational efficiency using several approaches. Adaptive mesh refinement introduces efficiency by varying the grid resolution in different parts of the model, using finer resolution for parts exhibiting greater spatial or temporal variability, such as the inner magnetosphere, and coarser resolution for parts with less structure, for example, in the solar wind or distant magnetotail. Meanwhile, high-order computational methods increase the precision of the numerical solution. The SWMF combines these methods for the global MHD model. In addition, we have developed the capability to embed kinetic models within the MHD model to address specific microphysical processes.
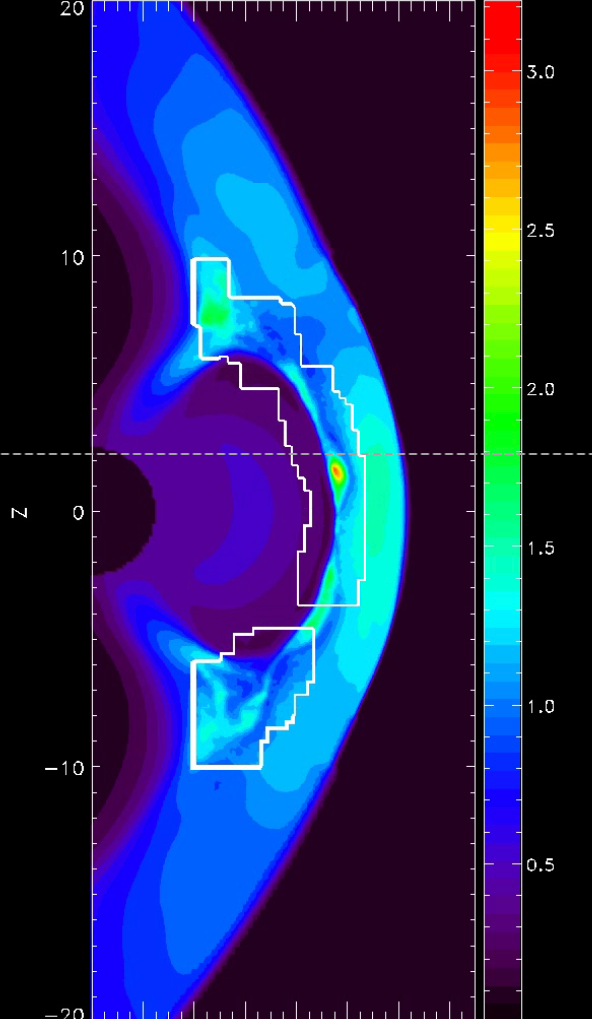
For example, magnetic reconnection, in which two different magnetic fields interact, causing field lines to come together and then violently split apart, is better represented with a kinetic-scale particle-in-cell (PIC) model (Figure 2). The PIC simulation can now be adaptively embedded into areas where the MHD model estimates that reconnection is most likely to occur. Similarly, models for the different ion species (most notably oxygen and helium) in the inner magnetosphere, plasmasphere, ionosphere, thermosphere, and the polar wind upwelling from the atmosphere, as well as models for the ring current and radiation belts that encircle Earth near and inside geostationary orbit, can all be coupled to the global MHD model.
With such a model suite, the SWMF successfully reproduces large-scale dynamics of geomagnetic storms and substorms, including the enhancement and decay of the ring current as well as smaller-scale structured flows in the magnetotail and geomagnetic disturbances recorded on the ground.
The MHD model can also be extended, using variants of the MHD equations, to include desired features such as Hall effects (electrical voltages induced by magnetic fields), multiple ion species and plasma populations, separate plasma pressures parallel and perpendicular to the magnetic field, and even relativistic effects. These models have the advantage of being computationally faster than their fully kinetic counterparts, and they can be smoothly and consistently coupled with embedded kinetic models.
The electrically neutral atmosphere is also coupled to space plasma and space weather processes and in part contributes to the electrodynamics of the ionosphere. At the moment, the global ionosphere-thermosphere model (GITM), a separate model also developed at the University of Michigan that integrates the neutral and ionized atmospheres, can use SWMF results as input. We are developing a two-way coupler that will allow the GITM outputs to influence the global ionospheric and magnetospheric dynamics in the SWMF.
Adding Artificial Intelligence into the Mix
The Solar Storms and Terrestrial Impacts Center (SOLSTICE), hosted by the University of Michigan, brings the most recent developments in machine learning to space weather modeling, including statisticians, data scientists, and computer scientists as integral members of the research group.
The multidisciplinary interaction has required teaching space physics to machine learning experts and new concepts of machine learning to space scientists, a process that has proven challenging and time-consuming but rewarding. The students involved have been especially excited to learn the scientific approaches and practices in the two research areas.
Our initial goal with SOLSTICE, which was initiated in 2020, is to tackle the problem of short lead times offered by available remote observations of solar flares. Solar flares are a major source of hazardous energetic particles that reach Earth within tens of minutes. Our early results indicate that signatures of a major flare eruption may appear on the solar surface as much as 24 hours in advance (Figure 3). If this proves to be generally true and we can identify these signatures in an operational setting, we could give satellite operators and humans in space more time to take protective measures. In addition, findings from SOLSTICE have the potential to advance our understanding of complex solar physics related to the formation of the large eruptions, illustrating how responding to operational needs can contribute to fundamental science.
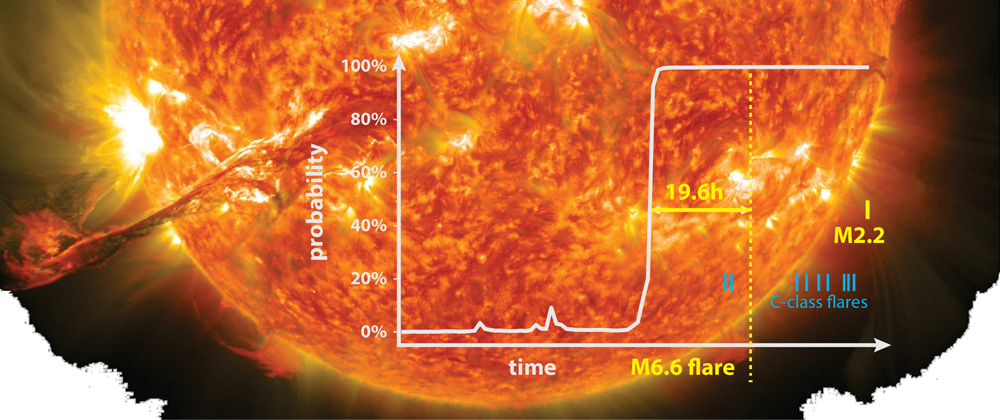
Models in Service to Society
In 2016, following a competitive selection process, SWPC selected the SWMF geospace model for operational use to issue warnings and short-term space weather predictions. The products and the interface to the model became available in October 2016; version 1.5 was put into operation in November 2017, and version 2.0 of this model began operations on 28 January 2021. SWPC currently has about 60,000 users, including satellite operators as well as energy system and power distribution companies. The energy sector is especially interested in local and regional geomagnetically induced currents, which arise because of strong and variable currents in the ionosphere.
The road from improving a research-based model to implementing a robust new version of the operational code is long and slow. Maintaining an operational code requires that it be efficient, accurate, robust, and user-friendly, including for nonexpert users. It must also be able to handle real-time input data with their accompanying errors. As both models and physical understanding are rapidly evolving, tight coupling between model users and researchers is necessary to guarantee the quality of the code and its predictions.
One of the most difficult questions that users pose is how accurate large-scale geospace models are (Figure 4). Although model output can be compared with local observations to check accuracy, such observations can only help us assess the performance of a small snapshot of the model within the entire geospace region. NASA and the National Science Foundation (NSF) have responded to the need to address uncertainties in models of the space environment by funding a series of efforts under a program called Next Generation Software for Data-driven Models of Space Weather with Quantified Uncertainties (SWQU).
An open-source version of the SWMF developed under the auspices of SWQU, called the NextGen Space Weather Modeling Framework, focuses on combining our Sun-to-Earth simulations with assimilation of both real-time and past data and uncertainty quantification. Data assimilation has the potential to significantly improve model performance, as has been shown by advances in terrestrial weather forecasting. The final model output will provide a probabilistic forecast of space weather impacts such as local geomagnetically induced currents (Figure 4).
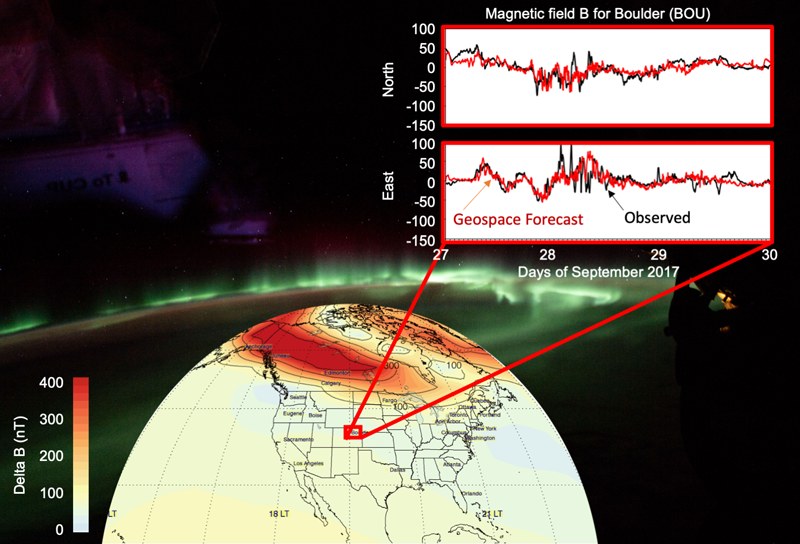
For operational applications it is important that the models can run fast enough to keep up with the incoming observations (ensuring, for example, that simulating 1 hour of physical time takes less than 1 hour of computing time). To speed the simulations to that level, the most computationally expensive parts of the model will need to run efficiently on current and future supercomputers employing graphical processing units (GPUs) in addition to traditional multicore CPUs.
The main product of this project will be the Michigan Sun-to-Earth Model with Quantified Uncertainty and Data Assimilation (MSTEM-QUDA), which now constitutes a major part of the SWMF.
It Takes a Village to Raise a Model
Development of the SWMF started in about 1990, and the model now comprises more than 1 million lines of code. From the beginning, the effort has included many researchers as both model users and developers. Feedback in this collaboration has led to significant scientific progress and has been critical to model development.
The full SWMF remains available via registration under a user license. In response to the current trend within funding organizations like NASA and NSF of publishing codes as open source, we have released MSTEM-QUDA on Github under a noncommercial open-source license, allowing other researchers to download, modify, and distribute it to fit individual needs.
A model of the upper atmosphere and ionosphere, called Aether, is being developed concurrently with MSTEM-QUDA as a community effort. This effort includes development of educational resources on coding and model development as well as a summer school for early-career researchers and offers opportunities for researchers to customize the model for different experiments. The goal is for the same model to be used for a variety of purposes, from addressing cutting-edge upper atmospheric, planetary, and exoplanetary science questions to prediction of the upper atmospheric state for satellite trajectory estimation and collision avoidance.
Although moving to open source is likely to lead to the desired outcome of increasing the user base for SWMF, the transition has highlighted the need to support the work to develop and maintain the code. The framework requires continuous development as computational methods and physical understanding evolve. Moreover, maintaining the modeling framework requires about 400 test runs on a nightly basis to ensure that the codes perform as expected. It is encouraging that funding agencies have started to recognize the need to support not only the scientific application of models but also the maintenance and development of modeling frameworks and computing environments.
Such efforts require collaboration among space scientists, mathematicians, numerical and computer scientists, and the space weather user community. SWMF user meetings gather about 100 users and developers to discuss recent advances in both model development and space science, and we are actively seeking new collaborations, including with algorithm and model developers as well as model users for both research and operational purposes.
Space weather poses substantial risks to humanity and has been identified as one of only two natural hazards that can affect the nation as a whole, the other being a pandemic. With ongoing and growing commitment from researchers, the user community, and funders, we can respond to society’s needs for accurate, reliable, and real-time space weather forecasting.