Data visualisation for decision making under deep uncertainty: current challenges and opportunities
This study explores the role of data visualization in decision-making under deep uncertainty (DMDU), a growing discipline tackling complex socio-environmental challenges, such as climate impacts and adaptation, natural resource management, and preparedness for extreme events. The role of visualization for both analysis (or exploratory) and communication (or explanatory) purposes, including engagement with stakeholders and the public, is discussed. A gap is identified in comprehensive guidelines on how visualizations are currently utilized and their potential in enhancing DMDU processes. Drawing on literature and insights from a recent workshop, we identify key challenges DMDU analysts face when visualizing data: managing complexity and dimensionality, effectively communicating uncertainty, and ensuring user engagement and interpretability. WA research agenda is proposed to address these challenges, by taxonomizing and evaluating the effectiveness of different visual forms in decision-making contexts and fostering interdisciplinary collaboration. It is argued that, through these efforts, the communication and usability of DMDU analyses can be improved, ultimately aiding in more informed and adaptive decision-making in the face of deep uncertainty.
Future takeaways begin with the initial step of classifying common data visualizations as fundamental components of DMDU analysis. Expanding on existing frameworks, cataloging and evaluating how different data visualizations facilitate both exploratory and explanatory processes in DMDU could enhance and promote their appropriate use. A second, and arguably more vital step, involves determining whether uncertainties are effectively communicated between analysts and decision-makers and are sufficiently incorporated into their reasoning processes. To achieve this, DMDU analysts could adopt methods from cognitive science scholars and formally assess how various visualizations enhance decision-making under deep uncertainty. Lastly, experts in DMDU and complex systems are typically proficient in the principles of transdisciplinarity and understand the importance of integrating diverse perspectives and methods into their work. It is crucial to capitalize on this openness and engage scholars from the domains of actionable knowledge, cognition, graphical inference, and the visualization of uncertainty and large complex data sets.
Explore further
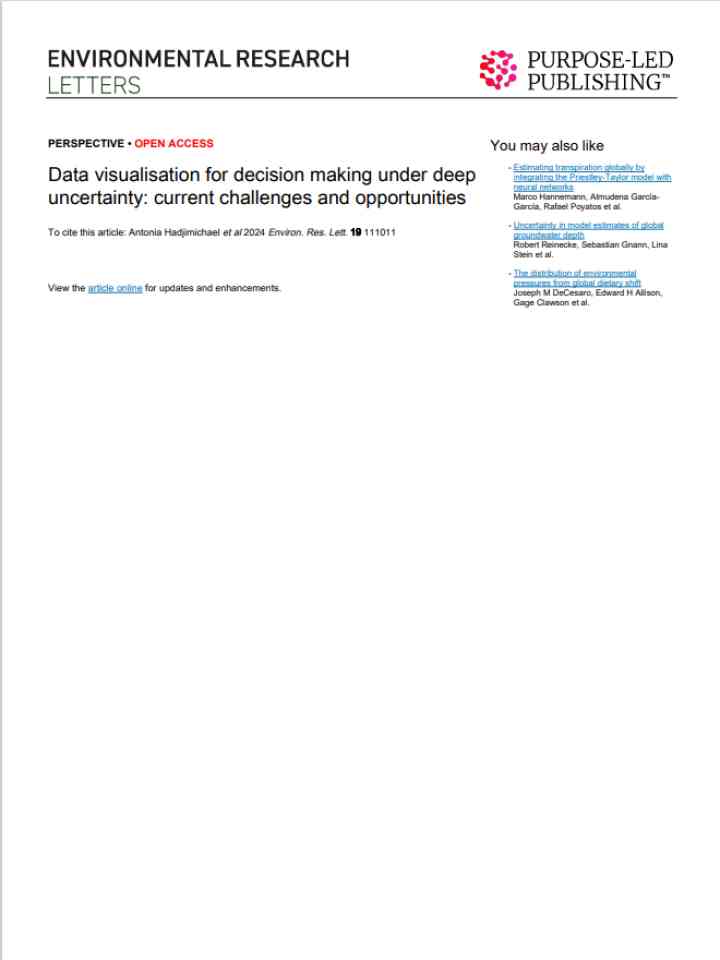