A deep learning technique based flood propagation experiment
This work presents an experiment involving detailed fluvial flood propagation process. As a type of disaster, floods have a high probability of occurrence, cover wide ranges, and can cause great harm, including casualties and the loss of property. Therefore, studies of flood propagation are of great significance for flood control and disaster reduction. The experiment described here considers a propagation process in which a fluvial flood flows through an urban river channel represented by a physical model, and three unsteady inflows of varying magnitudes are considered.
This paper presents an experimental data set for the flood propagation of a river channel, and the data can be used for the validation of numerical models designed for the simulation of fluvial floods. The available measurements of flood propagation consist of records of the spatial evolution of inundated areas and water levels at gauges of interest during the propagation process. The evolution of water levels can be recorded without disturbing the flow locally because the gauges are buried under the river channel in advance. The image processing method based on deep learning is nonintrusive and accurately shows the spatial evolution of inundated areas throughout the entire river channel. Moreover, the model can be reused and only needs to be trained once, thus possessing excellent potential for future use in flood propagation characterization. This approach provides a new and effective technical method for collecting data during physical experiments.
Explore further
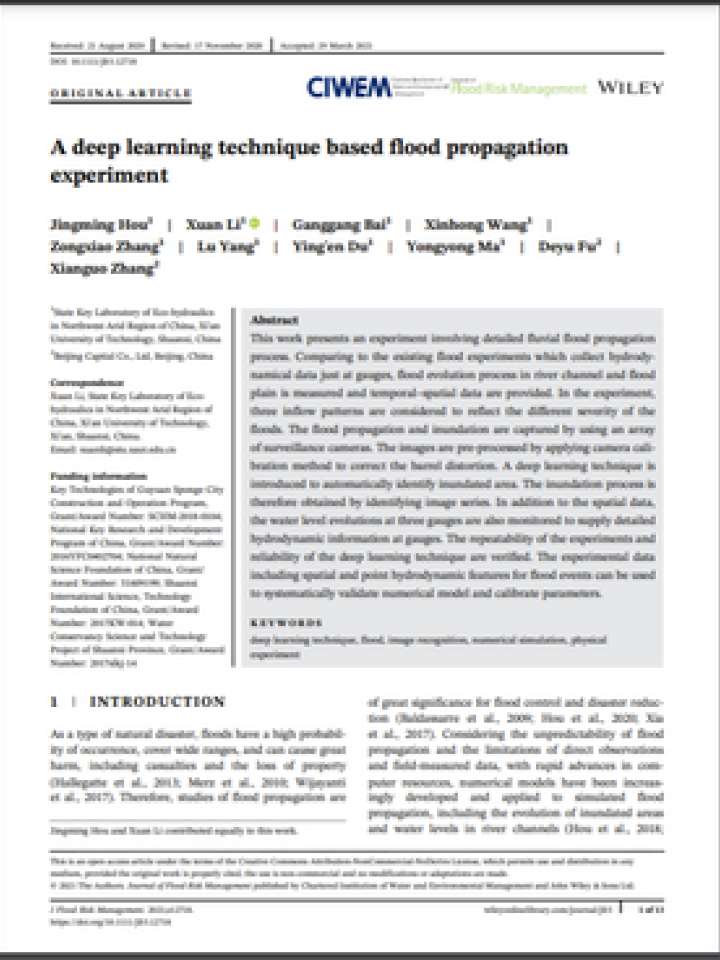