Enhancing flood susceptibility mapping in Meghna River basin by introducing ensemble Naive Bayes with stacking algorithms
This article intends to assess flood susceptibility mapping in Meghna River basin (MRB) and identified flood susceptible regions using three benchmark models including random forest (RF), support vector machine (SVM) and bagging with Naïve Bayes (NB) stacking ensemble algorithms (e.g. RF-NB; SVM-NB and Bagging-NB). The flood sample was partitioned into a training set (70%), and a validation set (30%), and the capability of prediction of flood-influencing variables was quantified by the multi-collinearity test. Several statistical metrics and Area Under the Receiver Operating Characteristics (AUROC) technique were applied to evaluate the techniques’ performance and precision.
The outcomes showed that the significant factors influencing flash floods include rainfall, distance from the river and river density. The NB-Bagging outperforms (≈ a prediction accuracy of 95.1%) than other models in predicting the risk of flooding in the MRB. Results obtained from NB-Bagging showed that 12% and 21% of the basin were demarcated as having high and very high flood susceptibility, respectively. This article identified that rainfall and distance from the river were the two most driving factors influencing flooding in the MRB. The present work will aid decision-makers and local authorities determine flood conditioning problems and efficient flood risk management to lessen the consequences.
Explore further
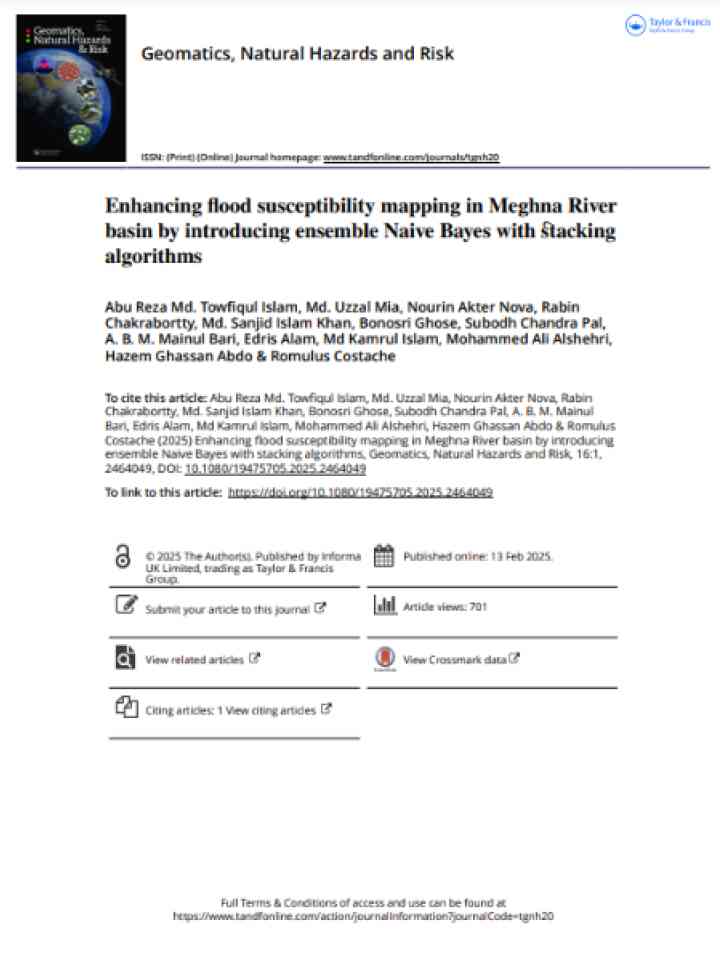