Rethinking predictive analytics for disaster resource allocation
This paper demonstrates the capacity of the the Integrated Internal DIsplacement Population Sampler (IIDIPUS) statistical engine code to inform disaster resource allocation on the short to mid-term. Informed disaster management requires detailed knowledge of the affected environment. Predictive analytics can help to provide such insight. The primary aim of IIDIPUS is to estimate human displacement, not damaged assets. This shift in focus increases the expected accuracy in the prediction of the spatial distribution of displacement, highlighting displacement hotspots. Sub-national and national vulnerability, sequential event modelling, and satellite image-based building damage assessment data are integrated into IIDIPUS.
This paper found that the IIDIPUS code predictions were more than 10 times more accurate in predicting the number of displaced people than two world renowned risk models: the Global Disaster Alert and Coordination System alert score and the United States Geological Survey PAGER risk score, over 101 historical earthquakes in 37 different countries. Mid to long term temporal displacement predictions utilise mobile phone data-based displacement information, with the potential for disaggregation by sex and age. Finally, coupling IIDIPUS with OpenRouteService facilitates the optimisation of the location and capacity of emergency shelters.
Explore further
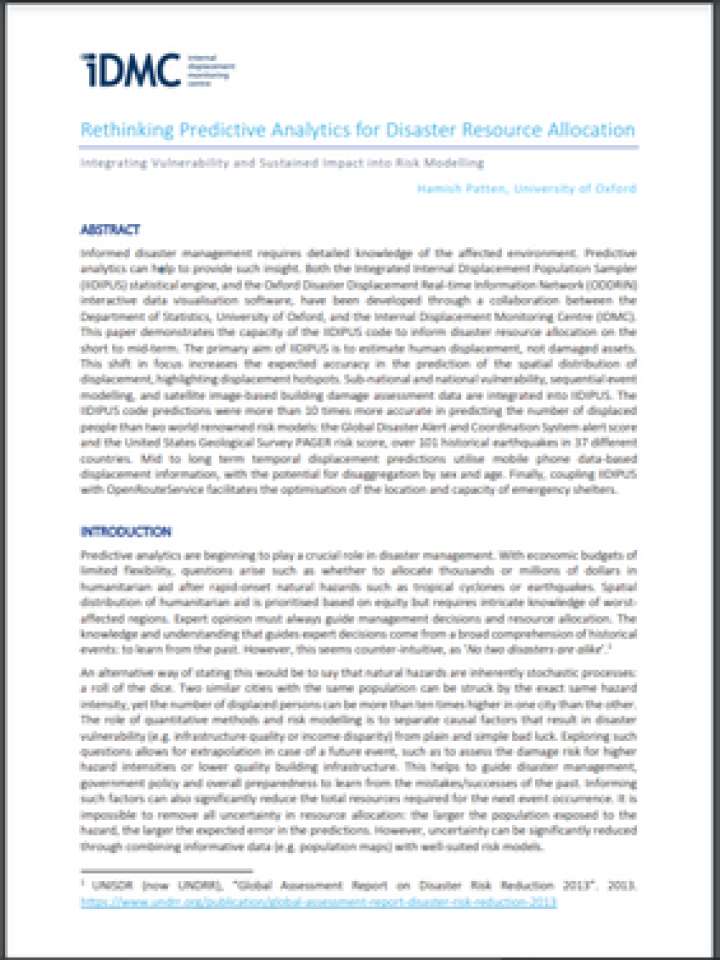