Weather radar nowcasting for extreme precipitation prediction based on the temporal and spatial generative adversarial network
This paper evaluates a proposed weather radar nowcasting method based on the Temporal and Spatial Generative Adversarial Network (TSGAN). This method can obtain accurate forecast results, especially in terms of spatial details, by extracting spatial-temporal features, combining attention mechanisms and using a dual-scale generator and a multi-scale discriminator. The nowcasting of convective weather, especially the nowcasting based on weather radar data, plays an essential role in meteorological operations for disaster prevention and mitigation. However, the drawback of most of the current forecasting methods based on deep learning suffer is that the forecast results become increasingly blurred as the forecast time increases.
The study finds from the case studies that the Generative Adversarial Network (GAN) method performs well in terms of forecast accuracy and spatial detail representation compared with traditional optical flow methods and popular deep learning methods. Therefore, the GAN method proposed in this study can provide strong decision support for forecasting heavy precipitation processes. At present, the proposed method has been successfully applied to the actual weather forecasting business system.
Explore further
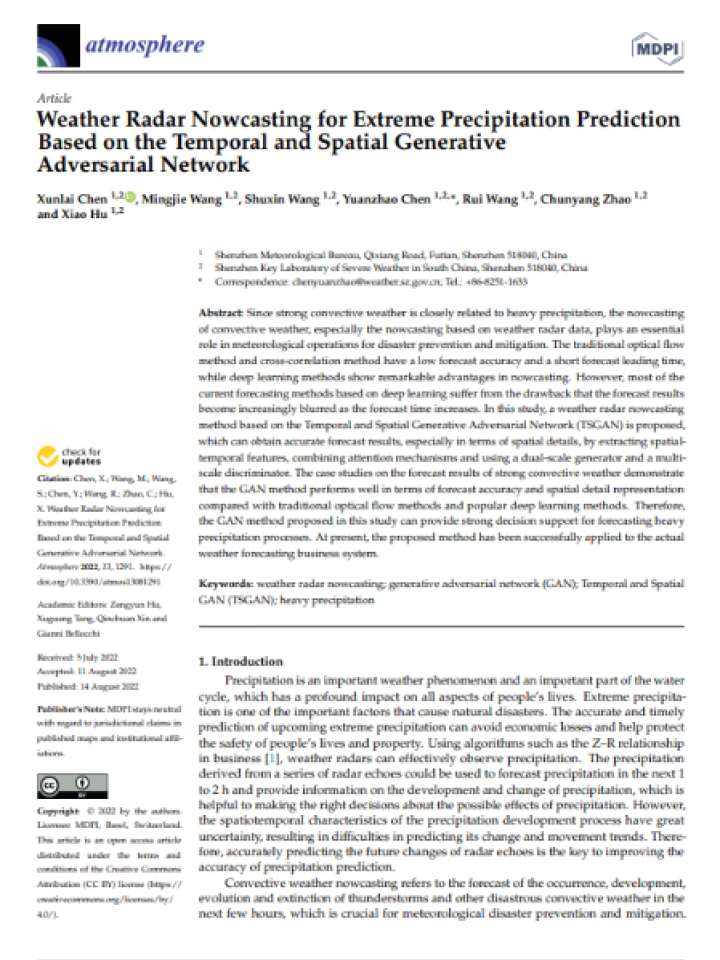