Advanced tools reveal critical infrastructure connections and help mitigate disasters
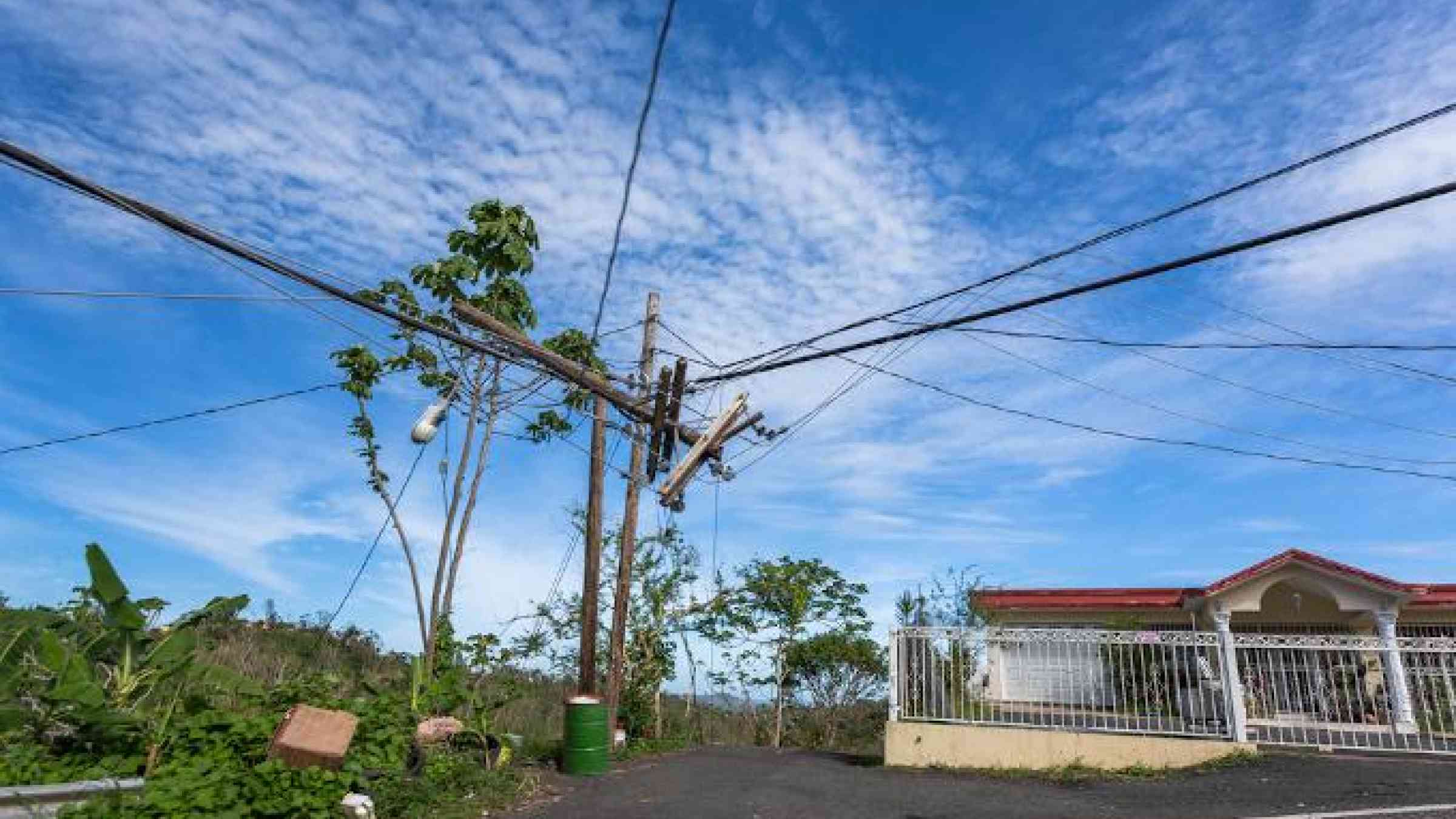
By John Spizzirri
Major disasters, whether natural or human-caused, most often have a devastating impact on local infrastructure that can result in the interruption of humanitarian efforts and deal an economic blow to communities large and small. No matter where you are in the world, these infrastructures are intimately linked, so that the failure of one can have a cascading effect on others.
Unfortunately, the details of how they’re interconnected isn’t always fully mapped out, making it hard for communities to determine which to bring online first after a disaster. However, Argonne is developing resources to help make the connections between the electrical grid and other lifeline infrastructure — natural gas, clean water, healthcare, communications and so on — and aid in those critical and timely decisions.
One disaster in particular may have provided the impetus for such a tool.
Shortly after Hurricane Maria tore through Puerto Rico in 2017, researchers from the U.S. Department of Energy’s (DOE) Argonne National Laboratory joined the recovery effort to help identify and prioritize those critical infrastructure systems that would benefit most from improvements in security and resilience.
The team developed the Puerto Rico Infrastructure Interdependency Assessment (PRIIA) tool to map connections between interdependent infrastructure — all of the various systems that basically support society, notes Josh Bergerson, principal infrastructure analyst in Argonne’s Decision and Infrastructure Sciences (DIS) division and a member of the PRIIA team.
Bergerson and other members of that team are continuing to advance the PRIIA technology through Argonne’s Laboratory Directed Research and Development (LDRD) program, but this time, the focus is on electricity-specific infrastructure and adding optimization and artificial intelligence (AI) components to reduce complexity and time-to-solution.
“The purpose of the research is to take the work that we started with FEMA (Federal Emergency Management Agency) using the PRIIA tool and think about ways we could augment and optimize our approach to modeling all the connections between interdependent infrastructure,” said Lawrence Paul Lewis, DIS program lead for technology implementation.
Softening the domino effect
Infrastructure systems such as energy, water, communications and transportation are interconnected; dependent on another such that the operation of one system affects the operation of another.
Your house is a microcosm of larger infrastructure systems that are connected in one way or another, and all the things that can go wrong if one of those dependencies — services from a utility, for instance — is damaged.
Electricity is typically the first to go, and perhaps the most important. Without it, you lose lights, refrigeration, air conditioning or heating, if it’s electric. You might lose water if you’re on a well or lose access to Internet and television.
Now imagine what these cascading effects might look like on a local or regional level. Ask the citizens of Puerto Rico, who endured the full brunt of these cascading infrastructure failures for more than a year.
Where earlier work focused on getting utilities and other critical infrastructure back online, the new Argonne collaboration is looking at how things break and developing plans in advance of disruption events.
Disruptions are not caused solely by natural hazards like storms, wildfires or flooding. Other causes include physical attacks, where a person or group intentionally targets infrastructure components through physical force, and cyberattacks, which have become more frequent and can have serious implications for critical infrastructure.
The optimized PRIIA tool will help analyze a system and point out its vulnerabilities, allowing stakeholders to address them and reduce the impact of any future events, whether natural or human-caused.
“We’re looking at various aspects of all of these interconnected infrastructure systems to try and figure out the gaps and the weaknesses that really limit their resilience,” said Bergerson. “And resilience here refers to your ability to plan, anticipate, adjust and remain operational following some type of disruption.”
One of the major challenges has been in trying to come up with an accurate schematic of a region’s infrastructure dependencies. While much public data exist about individual systems, no one collects information on the actual connections between those systems — for example, which electric power substation supports a particular water system.
The only way to get that information is to physically go into the field and observe the physical system itself, which has proven an extremely inefficient way of gathering information, Bergerson admits.
“In Puerto Rico, we spent six months and got a couple of hundred dependency connections out of tens of thousands that are there,” he recalled.
Because no official or authoritative database for infrastructure dependencies exists, the team began to standardize and organize the infrastructure data they did have — much of it from Puerto Rico, some from datasets, such as Homeland Infrastructure Foundation-Level Data, an aggregation from the Department of Homeland Security (DHS) of all critical infrastructure datasets that are publicly available.
One of the key outcomes of the project is the development of what the team calls a hypergraph, a generic superset of all reasonable connections or layouts of infrastructure assets, accompanied by a high-level characterization of each asset.
“You sort of lay out this generic SimCity-looking plan of asset characteristics and how they connect to each other,” said Lewis. “Then, as we approach a real-world problem, we feed real-world data through this hypergraph and that’s how we can start to populate our model.”
“So that’s one of the tough things, but also one of the victories that will come out of this.”
Building resources to support AI applications
In its effort to more accurately identify the impact of cascading failures, the team began collaborating with colleagues in Argonne’s Mathematics and Computer Science division (MCS) to further improve the overall modeling process.
By incorporating complex multistage models and algorithms into the PRIIA tool, they hope to reduce the labor intensiveness of tracking an exponential number of dependencies; reduce uncertainties associated with large-scale infrastructure systems; and address the computational complexities of infrastructure interdependencies through models motivated by game theory. These enhancements will allow the team to apply this new tool far beyond Puerto Rico, for which it was originally developed.
“The team was using a tool that allowed them to simulate what happens if something impacts certain pieces of infrastructure,” said Sven Leyffer, senior computational mathematician in MCS. “What they couldn’t do was address the question, ‘Is that the worst case that could happen to my infrastructure?’”
“To answer that, they would have had to go through an exponential number of combinations devoted to finding out the worst case. We can do it by just solving a single, large-scale optimization problem.”
The focus of the MCS work is to identify where a cascade might start in a particular system and which asset or combination of assets would create the worst cascade should they fail. The goal of this analysis, then, is to improve the resilience of those specific dependencies, whether by providing backups, introducing redundancies or increasing security.
The next step for the project includes incorporating an AI application called machine learning (ML) to speed up the process. ML models often require a lot of training data, however, which are not readily available for cascading failures. By applying their expertise in optimization and simulation techniques, Leyffer and his group are helping to find the most impactful cascades directly, information that can then be used to generate training data for ML models in the future.
“An AI application will help us do a better job of simulating how a disruption or degradation of operation to an upstream piece of infrastructure, like a very important transmission substation, would cascade across all the other infrastructure,” suggested Lewis.
“That is a very computationally complex question to answer, but it is a key question utility owners or operators must face.”
The combined tools and methods are intended to create an approach that teams can apply during planning and hazard mitigation processes, or in response and recovery phases to help prioritize the many actions needed to restore systems as efficiently and properly as possible.