Machine learning model uses social media for more accurate wildfire monitoring
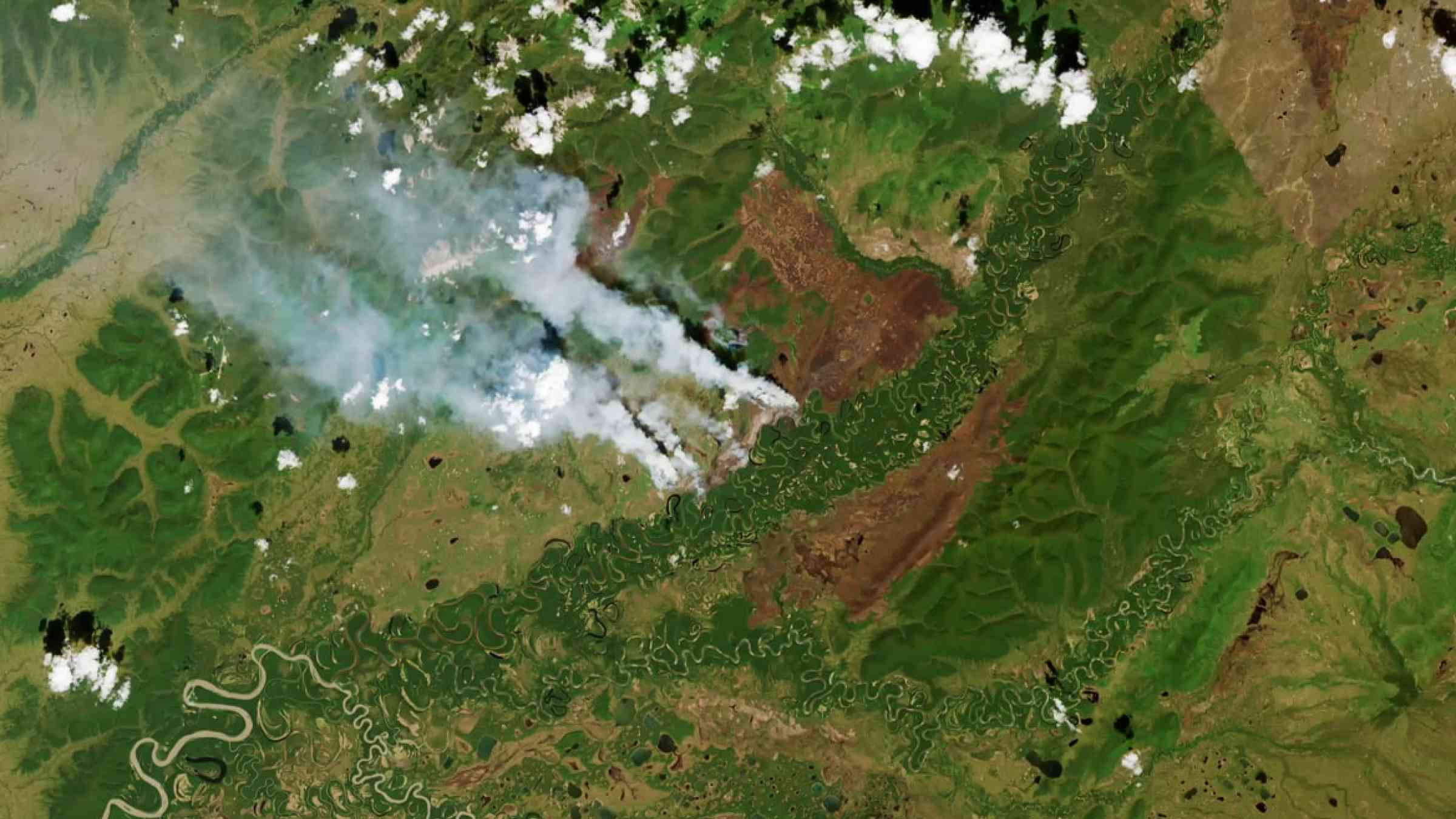
Scientists have developed a new machine learning model that uses social media data to predict and monitor wildfires more accurately in real-time.
Data scientists from Imperial’s Data Science Institute used machine learning - a subfield of artificial intelligence where computers learn from data and statistics, in a wildfire prediction model. In this new model, they combined social media data and geophysical satellite data to predict wildfire characteristics with high accuracy.
The study, published in the Journal of Computational Social Science, demonstrates how social media could be key to making more informed, socially driven decisions which could help disaster management teams to identify areas of immediate danger.
The intensity of wildfires and wildfire season length is increasing due to climate change, causing greater threats to populations worldwide. To control and mitigate the negative effects of wildfires, computational models exist that attempt to understand and predict the physical characteristics and evolution of these wildfire events.
"Social media is becoming increasingly important as a source of information and this work tries to make sense of it within the context of wildfires." Jake Lever
According to lead author, Jake Lever, who is a student of the Leverhulme Centre for Wildfires, Environment and Society: “Social media is becoming increasingly important as a source of information and this work tries to make sense of it within the context of wildfires. I hope this research will eventually make computational models more socially conscious, and therefore useful for disaster management teams.”
Real-time human sensors
The proliferation of social media in recent years has created a large amount of publicly available, unprocessed social data that captures the opinions and emotions of people in real-time. The immediate publishing of information on these platforms means that in disaster situations users act as ‘human sensors’, detecting and documenting events as they happen.
Increasingly, this social media data is being used by scientists for investigating, modeling, and mitigating natural disasters. Its widespread use during such events has helped develop disaster management applications from a humanitarian perspective, which is now being employed by aid agencies across the globe.
At present, traditional methods of sensing and predicting wildfires typically rely on geophysical sensors such as satellite data or remote sensing. However, this fails to accurately capture current wildfire information as it relies on lengthy simulations, satellite data which is timely to access, and it does not consider other social factors like blocked roads, downed powerlines, or evacuation orders.
According to Lever: “Instead of having a network of cameras or climate sensors to track a wildfire, you can use a network of social media users or ‘human sensors’ posting information about a disaster in real-time.”
“It's easier, cheaper, and quicker as you don’t have to go out and place a network of sensors and you can do it straight away from almost anywhere in the world.”
Sentimental Wildfires
By combining Twitter data with historical satellite data from the Global Fire Atlas, the team developed ‘Sentimental Wildfires’ – a machine learning model, trained on both social and physics wildfires data using a Sentimental Analysis.
Sentimental Analysis is a way of analysing emotional or subjective content in text, and it can be useful to help evaluate the levels of destruction in local areas, identify people or communities displaced, and improve overall disaster management and mitigation.
Previous research indicates that often regions that have a lower social sentiment (or higher amount of emotional or subjective content), correlate to a perceived disaster severity in that area.
In the study, the team tested the model with two 2016 datasets from the US and Australia. Their findings suggest that social media is a predictor of wildfire activity, and the numerical methods and algorithms proposed in the study can be applied to other natural hazard events in the future.
Next steps
The study represents a proof-of-concept for the socio-physical model for wildfire prediction, developed by Imperial’s Data Learning Group.
According to co-author Dr. Rossella Arucci: “The Data Learning Group at the DSI focuses on developing fundamental models for AI that are applied to different real-world applications, from air pollution to wildfires, economic models, social sciences and medicine.”
Moving forward, the team hopes to investigate how mis- and disinformation on social media can be accounted for by creating a more thorough verification process to extract the most meaningful and accurate information in natural disaster events.
In addition, combining data from multiple social media platforms will help to overcome any biases of using a single platform.
The model is also currently limited by local social media usage and internet connectivity. However, these problems will continue to decrease with social media usage continually rising and global internet connectivity on the horizon.