Development and implementation of a machine learning-based flood forecasting system in Kasese district, Uganda
This study focuses on developing a proof-of-concept prototype for a machine learning-based flood forecasting system in Kasese District, Uganda, a region frequently affected by flooding from the Nyamwamba River. Using a participatory design science approach, the researchers collaborated with local stakeholders, including students from the affected region, to identify system requirements. The project integrated hardware components like sensors and Arduino boards with software tools such as ThingSpeak for real-time monitoring and data visualization. The goal was to predict flooding based on key environmental variables—rainfall, temperature, and humidity—through machine learning algorithms (Linear Regression and K-Nearest Neighbours), and to send early warning alerts via SMS and social media.
The developed system successfully collected and analyzed real-time sensor data, enabling accurate flood level predictions using a linear regression model. It demonstrated cost-effectiveness and accessibility for rural Ugandan communities, relying on inexpensive hardware and leveraging mobile phone penetration for communication. The system predicted flood events based on sensor input, automatically alerting users when flood levels exceeded thresholds. However, the prototype was limited in scope, relying on a narrow set of climate indicators and requiring further validation. Still, the study offers valuable insights for implementing localized, data-driven early warning systems that enhance community resilience to flooding in Uganda and similar disaster-prone regions.
Explore further
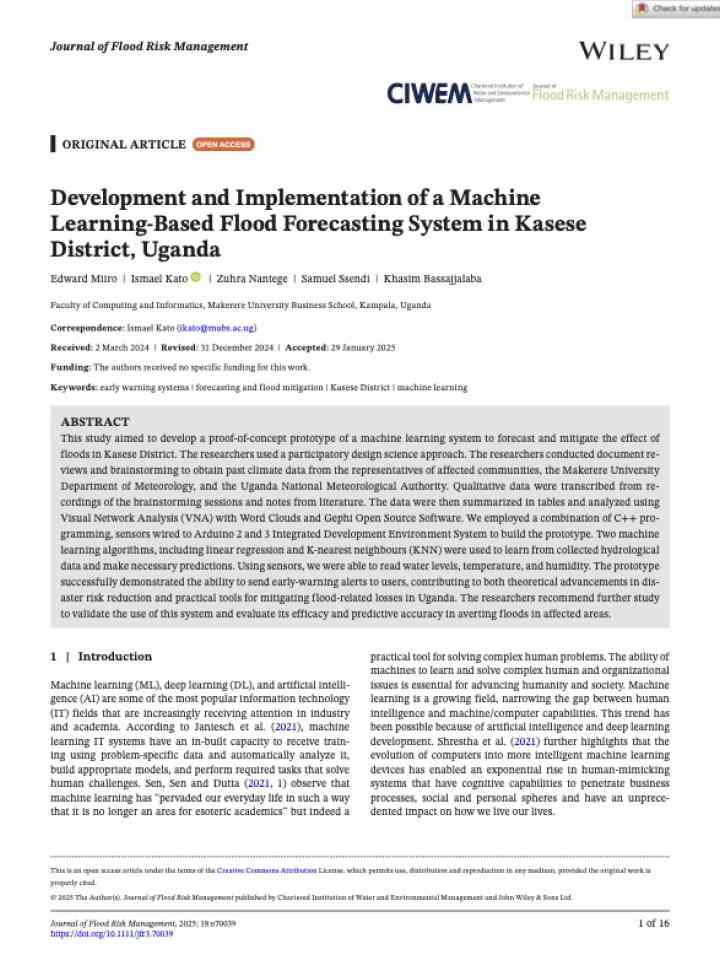