Predicting burn probability: Metamodels support strategic landscape decision making
This paper presents the development of an artificial neural network "metamodel" that can predict the burn probability outputs of computationally expensive process-based fire simulation models. The metamodel reproduces burn probability outputs with high accuracy and is much more computationally efficient (i.e., over 150 times faster) than process-based simulation models. The metamodel improves our ability to explore actions that reduce wildfire risk and develop effective strategies for landscape management. The metamodel has been incorporated into an interactive online tool, which can be used to test the effect of different actions on reducing fire spread across a case-study landscape.
By implementing effective strategies for developing Artificial neural network (ANN) metamodels, the study demonstrates that burn probability can be estimated accurately without repeated, costly simulations of individual fire events. This capability opens up new possibilities for studies that require numerous evaluations, such as optimizing fuel treatments. The integration of machine learning with existing models enhances the ability to explore trade-offs among fuel treatment options, supporting well-informed, balanced decision-making in wildfire management.
Explore further
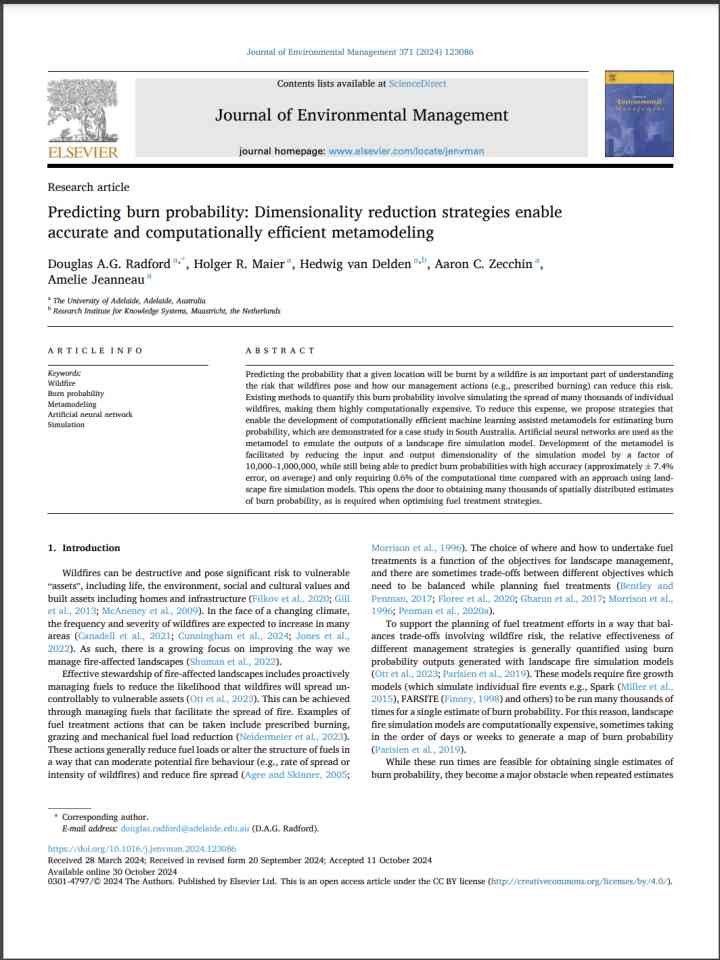